CDEI - AI Governance Report
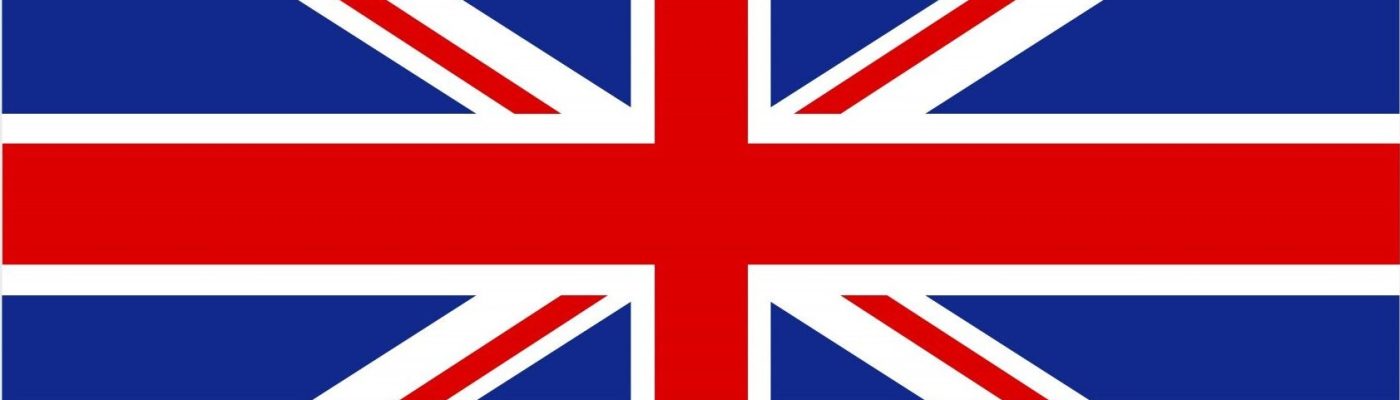
In April 2022, the Centre for Data Ethics and Innovation published its report on the governance expectation of AI, titled “CDEI- AI Governance” . This report assesses the publics perception of AI, and also highlights a range of real world application of AI alongside key governance frameworks.
This effort is intended to guide the development of policies and practices that ensure responsible and ethical AI use while fostering public trust.
Table of Contents
Introduction & Key Findings
Public Perceptions of AI
Awareness and Familiarity
Benefits and Risks
Real-World Applications of AI
Recruitment Processes
Mental Health Chatbots
HMRC Tax Fraud Detection
Shopping Suggestions
News Recommendations
Implications for AI Governance
Transparency
Fairness
Accountability
Conclusion
1. Introduction & Key Findings
The Center for Data Ethics and Innovation (CDEI) conducted an in-depth study on public perceptions and governance expectations of artificial intelligence (AI). This research aimed to align AI governance with the principles of transparency, fairness, and accountability. Key findings include:
Limited public awareness of complex AI applications.
Benefits of AI, such as efficiency and accessibility, outweigh risks in many contexts.
Strong public demand for AI governance to proactively address privacy, transparency, and fairness.
2. Public Perceptions of AI
Public understanding of AI remains narrow, often limited to low-risk applications like recommendation systems and virtual assistants. More advanced uses, such as decision-making in public services, are less understood. Media representations heavily influence perceptions, often framing AI as futuristic or overly intrusive.
Benefits and Risks
Perceived Benefits:
Enhanced societal efficiency and safety (e.g., crime detection, reducing human bias).
Increased accessibility for vulnerable groups, such as individuals with disabilities.
Key Concerns:
Invasion of privacy through data collection.
Over-reliance on AI leading to societal detachment and health issues.
Potential manipulation of public opinion via biased algorithms.
3. Real-World Applications of AI
Recruitment Processes
AI in recruitment is appreciated for its ability to reduce human bias but raises concerns about:
Transparency: Clear criteria for candidate selection are essential.
Fairness: Participants demand that irrelevant factors like ethnicity or gender be excluded.
Accountability: Unsuccessful candidates expect detailed feedback and the option to challenge decisions.
Mental Health Chatbots
Public trust in mental health chatbots hinges on:
Transparency: Users must know they are interacting with AI and have access to human support if needed.
Fairness: Personal data such as mood and health history is deemed critical for effective support.
Accountability: Mechanisms to escalate concerns and provide feedback are non-negotiable.
HMRC Tax Fraud Detection
The use of AI to flag tax fraud is seen as beneficial but demands:
Transparency: Clear communication about the data sources and decision-making criteria.
Fairness: Strict adherence to anti-discrimination laws.
Accountability: Easy access to human investigators for disputes.
Shopping Suggestions
AI-driven shopping recommendations are generally accepted but should:
Respect user privacy and allow data control.
Avoid irrelevant or intrusive data sources, like social media interactions.
News Recommendations
While the risks of AI in news curation are seen as low, users expect:
Transparency: Simple explanations of how recommendations are formed.
Fairness: Exclusion of sensitive personal data, such as recent conversations.
4. Implications for AI Governance
Transparency
Public expectations for transparency vary by use case. High-risk applications like tax fraud detection require detailed disclosure, while simpler cases, such as shopping suggestions, demand basic opt-in/opt-out options. Key priorities include:
Clear communication about the presence of AI in decision-making.
Accessible explanations of data use and decision-making processes.
Fairness
Participants emphasize:
Exclusion of irrelevant demographic data.
Equal treatment for all, with special considerations for vulnerable groups.
Stringent governance to minimize biases.
Accountability
High-risk scenarios necessitate robust accountability mechanisms, including:
Human intervention for error correction.
User-friendly systems to adjust preferences or contest AI decisions.
Continuous oversight and regular system audits.
5. Conclusion
The CDEI’s research highlights the critical role of public expectations in shaping AI governance. While public understanding of AI remains limited, the principles of transparency, fairness, and accountability resonate deeply. Policymakers must prioritize these principles, ensuring governance frameworks evolve alongside AI technology to maintain public trust and societal benefit.
If you would like to access other resources on the United Kingdom’s regulation of AI. You can access them here.